Challenges:
• Most existing vector extraction works focus on a specific target and are fragile to category variety;
• Especially Line- and polygon-shape objects are very different in topological structure.
Contributions:
• We propose an innovative class-agnostic model TopDiG,to directly extract topological directional graphs from remote sensing images regardless of target types.
• We design topology-concentrated node detector to extract nodes and corresponding features for both line-and polygon-shape targets.
• We conduct dynamic graph supervision strategy to facilitate inference in practice .
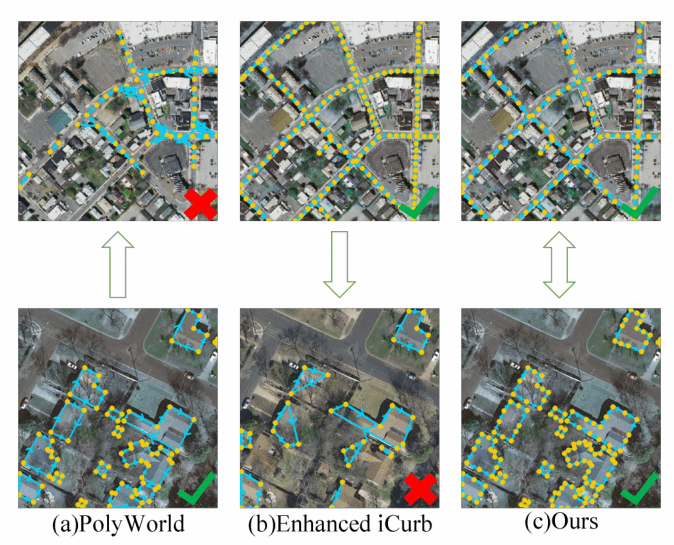
TopDiG can extract vector topology of both line- and polygon-shape objects
TopDiG formulates diverse vector topological structures as directional graphs and works by sequentially predicting target nodes and their connections.
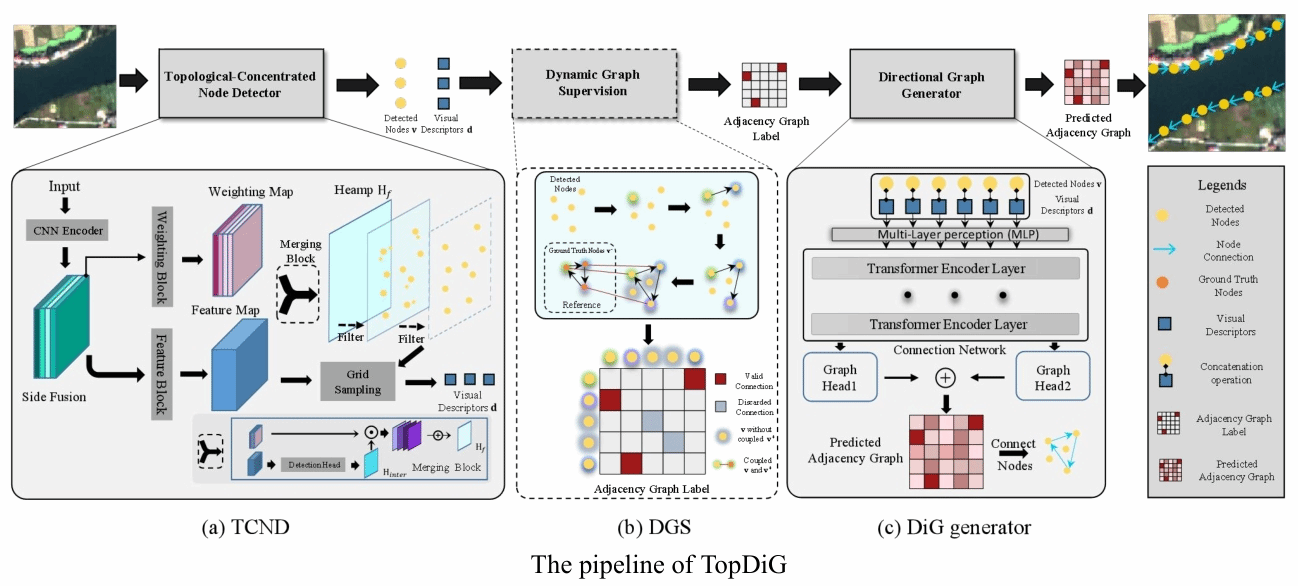
Topology-Concentrated Node detector(TCND):
• In satellite and aerial images, line shape objects can be seen as edges
• TCND minimizes sematic contexts and concentrates on low-level geometric features to achieve category independency.
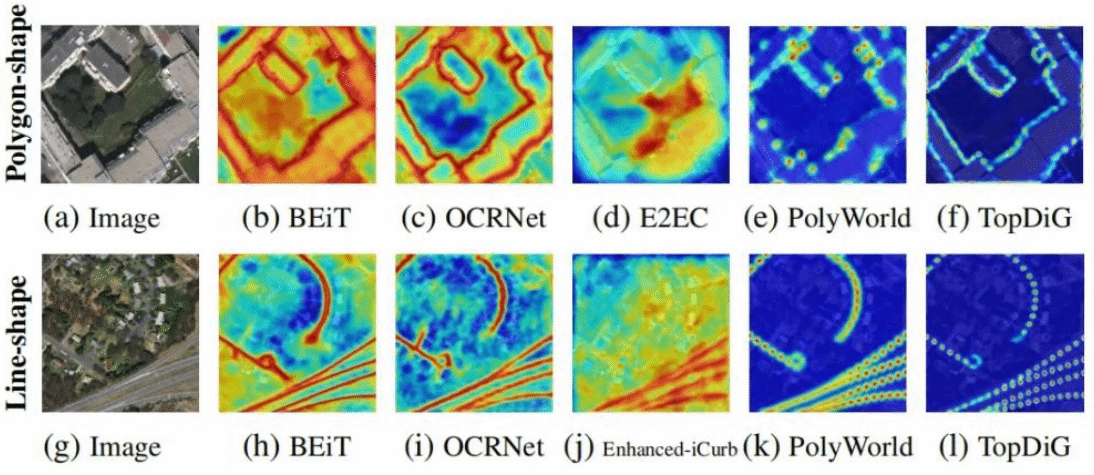
Visual comparison of attentive maps for a few methods
Dynamic Graph Supervision(DGS):
• Dynamically generates adjacency matrix labels according to real-time unordered predict nodes in each training.
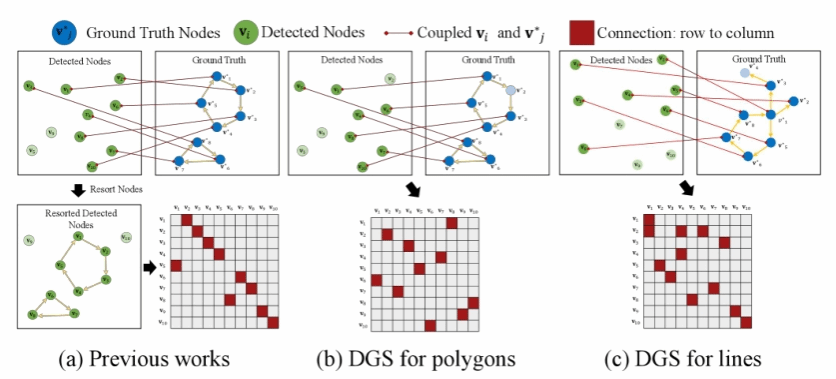
Visual comparison of the adjacency matrix label generation by previous works and DGS
Visual comparison:
• TopDiG performs better in topology quality and completeness, especially in concave/hierarchical polygon contours and parallel/crossing line object centerlines.
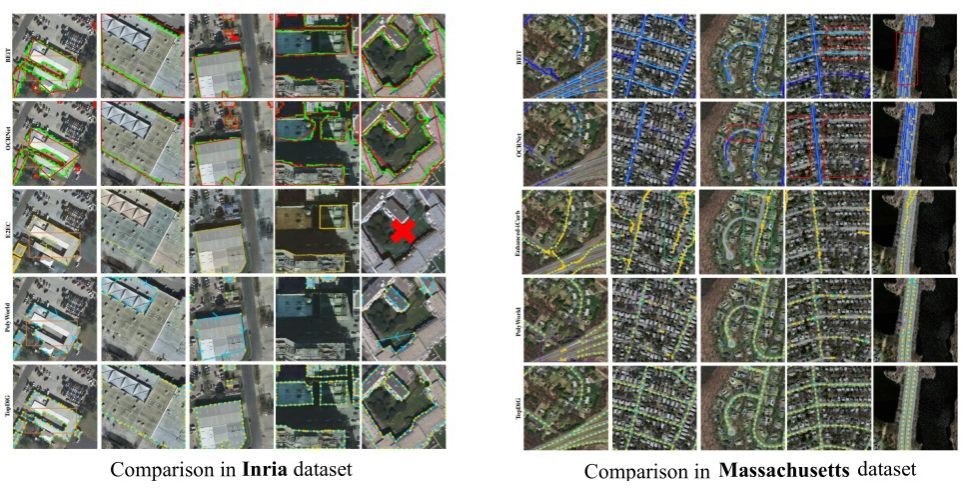
Quantitative comparison:
• TopDiG obtains competitive scores in pixel-wise metrics
• TopDiG achieve state-of-the-art performance in topology-wise metrics
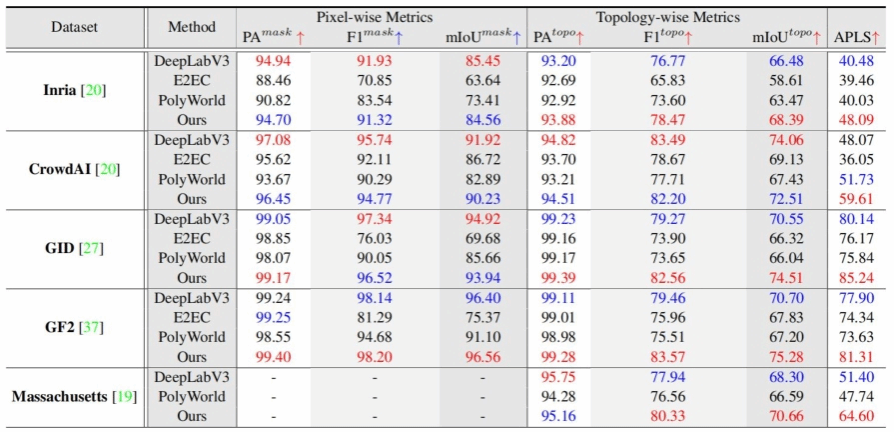